Endpoint AI is a new frontier in the space of artificial intelligence that takes the processing power of AI to the edge. It is a revolutionary way of managing information, accumulating relevant data, and making decisions locally on a device. Endpoint AI employs intelligent functionality at the edge of the network, in other words, it transforms the IoT devices that are used to compute data into smarter tools embedded with AI features. This in turn improves real-time decision-making capabilities and functionalities. The goal is to bring machine-learning based intelligent decision-making physically closer to the source of the data. In this context, Embedded vision shifts to the Endpoint. Embedded vision incorporates more than breaking down images or videos into pixels - it is the means to understand pixels, make sense of what is inside and support making a smart decision based on specific events that transpire. There have been massive endeavors at the research and industry levels to develop and improve AI technologies and algorithms.
What is Embedded Vision?
Embedded computer vision is a technology that imparts machines with the ability to see i.e., the sense of sight, which enables them to explore the environment with the support of machine-learning and deep-learning algorithms. There are numerous applications across several industries whose functionality relies on computer vision, thus becoming an integral part of technological procedures. In precise terms, computer vision is one of the Artificial Intelligent (AI) fields that enables machines to extract meaningful information from digital multimedia sources to take actions or make recommendations based on the information that has been obtained. Computer vision is, to some extent, akin to the human sense of sight. However, the two differ on several grounds. Human sight has the exceptional ability to understand many and varied things from what it sees. On the other hand, computer vision recognizes only what it has been trained on and what it is designed to do exactly, and that too with an error rate. AI in embedded vision processes trains the machines to perform supposed functions with the least processing time and has an upper edge over human sight in analyzing hundreds of thousands of images in a lesser timeframe.
Embedded vision is one of the leading technologies with embedded AI utilized in smart endpoint applications in a wide range of consumer and industrial applications. There are a number of value-added use cases examples; such as counting/analyzing the quality of products on a factory line, keeping a tally of people in a crowd, identifying objects, and analyzing the contents of a specific area in the environment to name a few.
Whilst considering the processing of embedded vision applications at the endpoint, the performance of such an operation may face some challenges. The data flow from the vision sensing device to the cloud for the purposes of analyzing and processing could be very large and may exceed the network available bandwidth. For instance, a camera with 1920 x1080 and operating with 30FPS (Frame Per Second) may generate about 190MB/S of data. In addition to privacy concerns, this substantial amount of data contributes to latency during the round trip of data from the edge to the cloud, then back again to the endpoint. These limitations could negatively impact the employment of embedded vision technologies in real-time applications.
IoT Security is also a concern in the adoption and growth of embedded vision applications across any segment. In general, all IoT devices must be secured. A critical issue and concern in the use of smart vision devices is the possible misuse of sensitive images and videos. Unauthorized access to smart cameras, for example, is not only a breach of privacy, but it could pave a way for a more harmful outcome.
Vision AI at the Endpoint
- Endpoint AI can enable image processing to infer a complex insight from a huge number of captured images.
- AI uses machine-learning and deep-learning capabilities within smart imaging devices to check a huge amount of previously well-known use cases.
- For optimum performance, Embedded vision requires AI algorithms to run on the endpoint devices and not transmit data to the cloud. The data here is captured by the imaging recognition device, then processed and analyzed in the same device.
The limitation of power consumption at the endpoint is still available, where the microcontrollers or microprocessors need to have more efficiency to take on high volumes of multiply-accumulate (MAC) that are required for AI processing.
Deployment of AI Vision Applications
There are unlimited use cases for the deployment of AI in vision applications in the real world. Here are some of the examples where Renesas can provide comprehensive MCU and MPU-based solutions inc. all the necessary SW and tools to enable quick development.
Smart Access Control:
Security access control systems are becoming more valuable with the addition of voice and facial recognition features. Real-time recognition requires embedded systems with very high computational capabilities and on-chip hardware acceleration. To meet this challenge, Renesas provides a choice of MCU or MPU that offers very high computational power that also integrates many key features that are critical to high-performance facial and voice recognition systems such as built-in H.265 hardware decoding, 2D/3D graphic acceleration, and ECC on internal and external memory to eliminate soft-errors and allow for high-speed video processing.
Industrial Control:
Embedded vision has a huge impact as it utilizes many applications, including product safety, automation, sorting of products, and many more. AI techniques can perform multiple operations in the production process such as packaging and distribution, which can ensure quality and safety during production in all stages. Safety is needed in areas such as critical infrastructure, warehouses, production plants, and buildings that require a high level of human resources.
Transportation:
Computer vision presents a large scale of ways to improve transportation services. In self-driving cars as an example, computer vision is used to detect and classify objects on the road. It is also used to create 3D maps and estimate movement around. By using computer vision, self-driving cars gather information from the environment using cameras and sensors, which then interpret and analyze the data to make the most suitable response by using vision techniques such as pattern recognition, feature extraction, and object tracking.
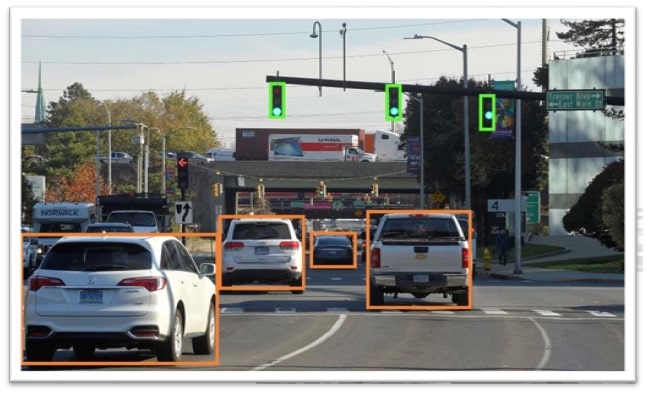
In general, Embedded vision can serve many purposes, and these functionalities can be used after customization and the needed training on different types of datasets from many areas. Functionalities include monitoring physical area, recognizing intrusion, detecting crowd density, and counting humans or objects, or animals. They also include identifying people or finding cars based on car numbers, detection of motion, and human behavior analysis in different cases.
Case study: Agricultural Plant Disease Detection
Vision AI and deep learning may be employed to detect various anomalies - plant disease detection is one example of this type of system. Deep learning algorithms -one of the AI techniques- are used widely for this purpose. According to research, computer vision gives better, accurate, fast, and low-cost results, as compared to the costly and slow labor-intensive results of previous methods.
The process that is used in this case study can be applied to any other detection. There are three main steps for using deep learning in computer/machine vision:
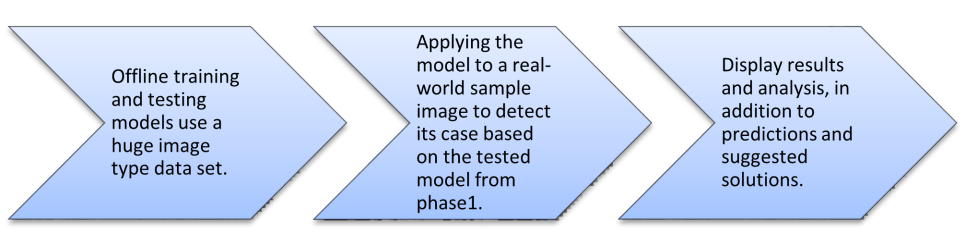
Step one is performed on normal computers in the lab, whereas step two is deployed on a microcontroller at the endpoint, which can be on the farm. Results in step three are displayed on the screen on the user side. The following diagram shows the process in general.
Conclusion:
We are experiencing a revolution in high-performance smart vision applications across a number of segments. The trend is well supported by the growing computational power of microcontrollers and microprocessors at the endpoints, opening up great opportunities for exciting new vision applications. Renesas Vision AI solutions can help you to enhance overall system capability by delivering embedded AI technology with intelligent data processing at the endpoint. Our advanced image processing solutions at the edge are provided through a unique combination of low power, multimodal, multi-feature AI inference capabilities. Take the chance now and start developing your vision AI application with Renesas Electronics.
Find information on products and technologies to help you implement AI solutions at renesas.com/AI.